Ongoing Research
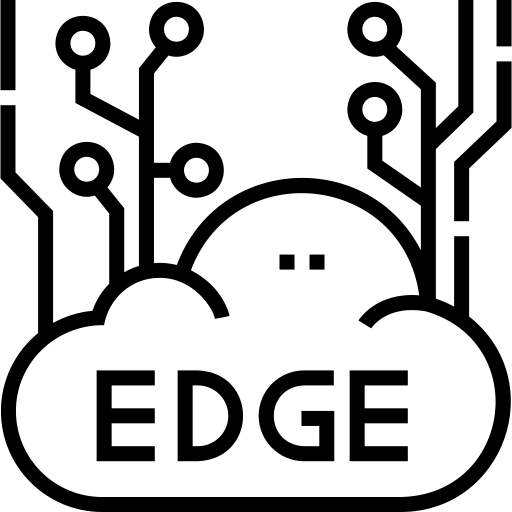
Practical ML for Edge Devices
Current Collaborators: Palantir, POSTECH, SNU, UIUC
The demand for realistic machine learning systems tailored for edge devices has grown in recent years. A number of learning paradigms well-suited for edge devices have emerged, such as Continual Learning and Federated Learning. However, the adoption of such paradigms has been difficult as prior works do not adequately address the growing demand for system efficiency in practical edge environments.
In response, our team has focused on system solutions for cost-effective on-device learning. We build systems that optimize the energy-accuracy trade-offs and adapt to dynamic resource states of the target devices.
Related Publications: REP, Miro, CarM
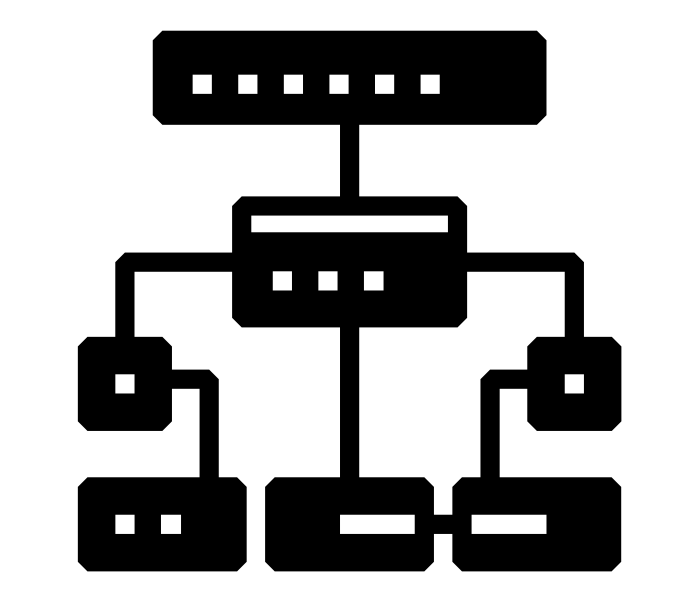
System Solutions for Large-scale ML
Current Collaborators: Azure Research – Systems, Microsoft Research Asia, Microsoft Research Redmond, Samsung Research, KAIST, UC Merced, USC
ML applications are increasingly requiring greater computing resources with the rapid growth in size of the state-of-art DNNs (e.g. LLMs). Many studies and productions have been released to address this problem.
We strive to provide system solutions for different phases of DNN workloads such as efficient model parallelization for GPU clusters, improving GPU utilization, efficient data loading and preprocessing for DNN.
Related Publications: Cascading KV Cache, HiP Attention, Metis, FusionFlow, EnvPipe, Sibylla, HUVM, Zico, Philly, Tiresias
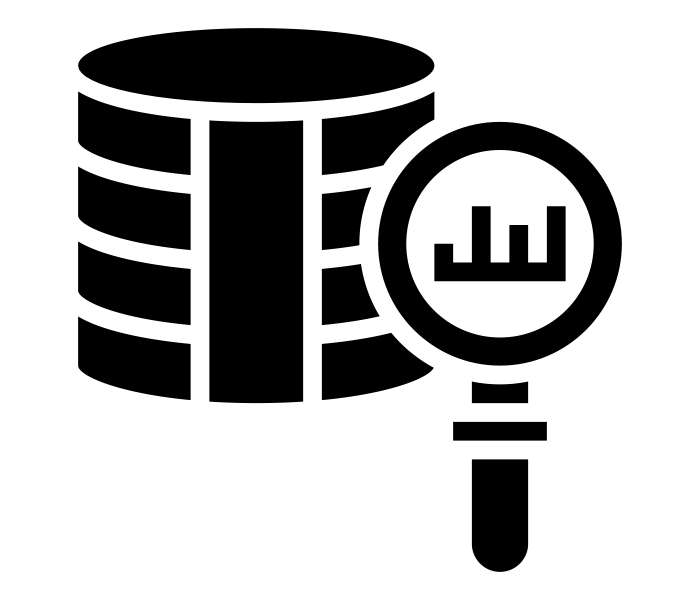
Fast and Scalable Big Data Analytics
Current Collaborators: Amazon, Samsung Electronics, SNU
Big data analytics plays a crucial role in today's data-driven world, offering the potential for improved decision-making, operational efficiency, and innovation across a wide range of domains.
We aim to build fast and scalable systems for real-time big data analytics at cloud/IoT scale that enable system operators to promptly troubleshoot system anomalies, improve the performance and reliability of their services, and optimize the performance tailored to specific workload characteristics.